Explore Black Box AI, its rise across industries like healthcare and coding, and the challenges of AI bias and transparency. Learn about Explainable AI (XAI), the debate on transparency vs. performance, and insights from experts. Discover how AI can balance accuracy and interpretability and why it's essential to upskill for the future of AI.
- 1. Introduction
- 2. Understanding Black Box AI
- 3. The Rise of Black Box AI
- 4. Challenges and Concerns
- 5. AI Bias Across Industries: The Hidden Numbers
- 6. Explainable AI: Shedding Light on the Black Box
- 7. Making AI Decisions More Transparent
- 8. Black Box AI in Coding
- 9. The Future of AI-Powered Coding
- 10. The Debate: Transparency vs. Performance
- 11. The Balancing Act: Accuracy vs. Interpretability
- 12. What Experts Are Saying
- 13. Can We Have the Best of Both Worlds?
- 14. Conclusion: Demystifying Black Box AI
- 15. FAQ: Your Questions About Black Box AI
Table Of Content
Introduction
Imagine asking your voice assistant, “What’s the weather like today?” and receiving a precise answer in seconds. Cool, right? But here’s the catch—how did the assistant arrive at that answer? What went on behind the scenes in its decision-making process? Enter Black Box AI, a fascinating yet mystifying area of artificial intelligence where systems generate results without offering any clarity about how they reached those conclusions.
In simple terms, Black Box AI refers to AI systems whose internal processes are invisible or incomprehensible to humans. You give input, get output, but the “how” remains hidden, wrapped in a metaphorical black box. This isn’t some fringe technology either, it’s everywhere. From predicting loan approvals and healthcare diagnoses to powering recommendation algorithms and autonomous vehicles, black box AI is silently running the world around us.
Why Black Box AI is Named So?
Black box AI gets its name because, like a literal black box, you can see the inputs and outputs but have no clear idea of what happens inside—its decision-making is a mysterious, impenetrable process.
Understanding Black Box AI
What is Black Box AI?
At its core, Black Box AI refers to artificial intelligence systems, especially ‘deep learning models’ whose decision-making processes are not easily understood or explained. These systems take in data, process it using complex algorithms, and generate outputs, but the exact steps they follow remain hidden, even to the developers who built them.
While traditional AI models, such as decision trees or linear regression, are transparent and their logic can be easily understood, Blackbox AI models operate in a way that is difficult to decipher. This lack of transparency is both a strength and a challenge, depending on the context in which it is used.
To put it simply, it’s like using a vending machine with no transparent panel. You insert money, press a button, and get a snack, but you have no clue about the internal mechanics that made it happen. Now, contrast this with White Box AI, which operates with full transparency, allowing users to see and understand the logic behind every decision.
White Box AI models are typically rule-based or use interpretable algorithms, making them more suitable for industries where explainability is critical, such as healthcare and finance.
How Does Black Box AI Work?
To understand how Black Box AI functions, let’s break it down into three key stages:
1. Data Input –The AI model receives raw data, such as images, text, or numerical values. This could be anything from customer purchase history to MRI scans.
2. Processing (Hidden Layers) – The data moves through multiple hidden layers within a deep learning model, where thousands (or even millions) of parameters adjust and interact to detect patterns and make predictions.
3. Output – The AI generates a result, such as identifying a cat in an image, approving or rejecting a loan, or recommending a product. However, the logic behind why it arrived at that decision remains concealed.
Here’s a simple flowchart illustrating the process:
Data Input
↓
Hidden Processing Layers
↓
Final Output
One major reason why Black Box AI is difficult to interpret is that these hidden layers continuously adjust based on incoming data. Unlike traditional rule-based systems, which follow clear "if-then" logic, deep learning models tweak their parameters autonomously, making it nearly impossible to trace how a specific decision was made.
Fun Fact-
Hidden Layers Mimic Biological Brains
Black box AIs like deep neural networks are often modeled after biological neural networks. Surprisingly, certain patterns in these AI systems resemble brain activity observed in
humans during problem-solving tasks, such as visual or language recognition.
The Rise of Black Box AI
Historical Context
AI evolved from rule-based systems to machine learning and deep learning in the 2000s. Early AI struggled with complexity, but breakthroughs like Geoffrey Hinton’s 2012 deep learning for image recognition led to the rise of Black Box AI. These powerful models learn from massive data sets but are difficult to interpret due to their complexity.
Current Applications
Today, Black Box AI is deeply integrated into multiple industries, influencing decisions in ways many people don’t even realize. Here are some key areas where it plays a crucial role:
- Healthcare 🏥 – AI-powered diagnostic tools analyze medical scans, predict diseases, and suggest treatments. For example, deep learning models can detect cancer in X-rays with remarkable accuracy.
- Finance 💰 – AI algorithms assess credit scores, detect fraud, and automate stock trading.
- Autonomous Vehicles 🚗 – Self-driving cars rely on deep learning models to recognize objects, make split-second driving decisions, and navigate roads.
- Retail & E-commerce 🛍️ – Recommendation engines, like those used by Amazon and Netflix, suggest products and content based on user behavior.
- Law Enforcement & Surveillance 🔍 – AI-powered facial recognition and predictive policing are increasingly used to identify suspects and prevent crime.
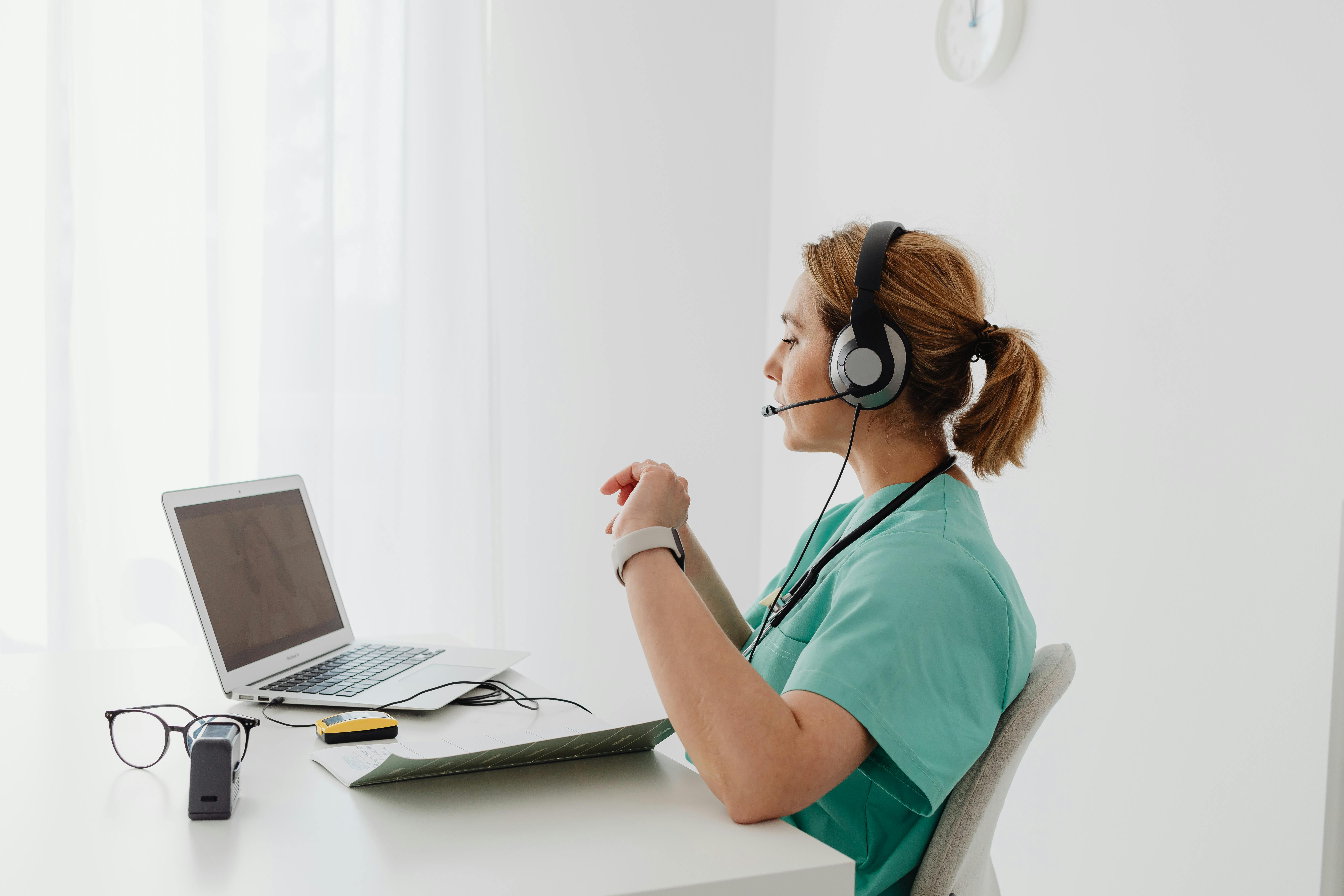
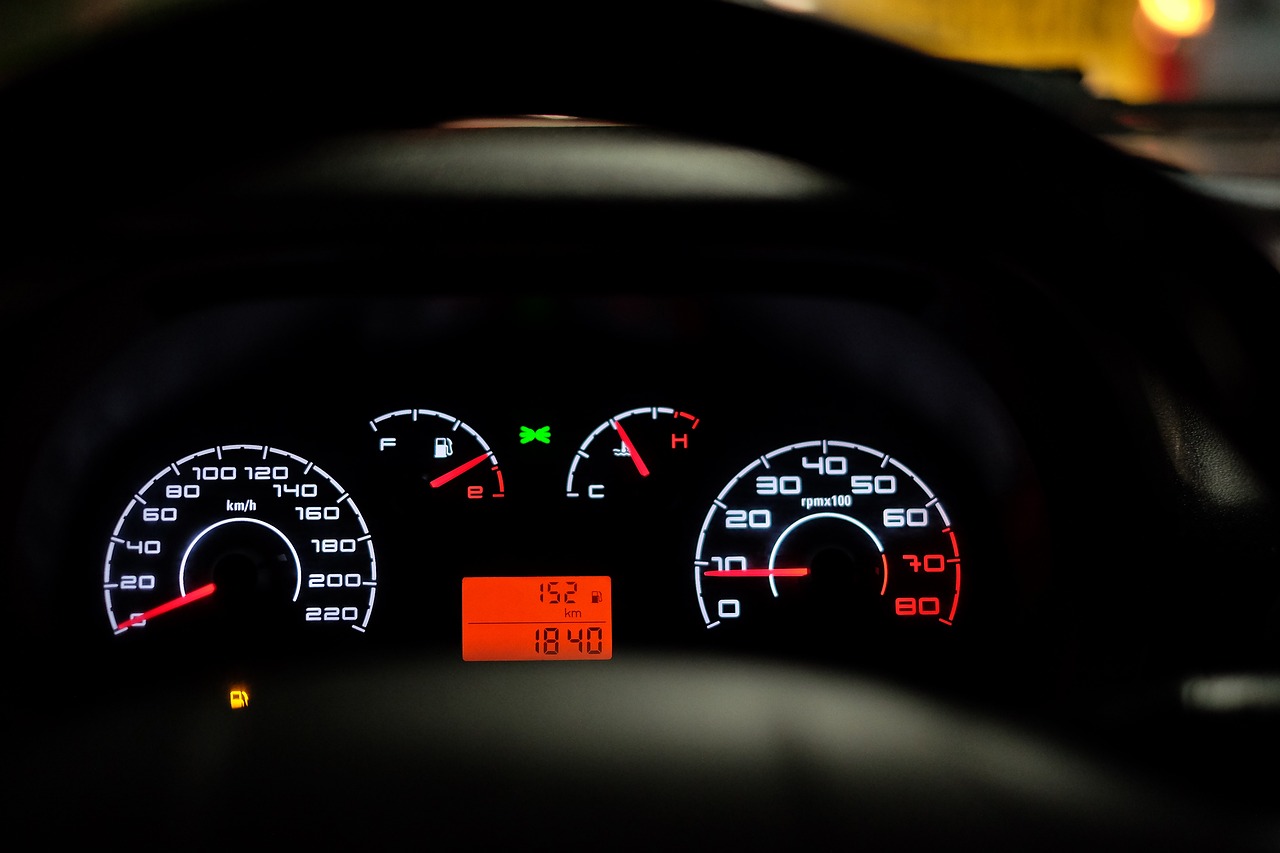
Fun Fact-
Some Black Boxes "Forget" What They've Learned
When neural networks undergo catastrophic forgetting, they lose knowledge about previously learned tasks while adapting to new ones.
Challenges and Concerns
As powerful as Black Box AI is, it comes with a fair share of challenges some of which can have serious real-world consequences. From lack of transparency to ethical dilemmas, these issues have sparked debates among researchers, policymakers, and everyday users alike. Let’s break them down.
1. Lack of Transparency 🕵 ♂️
One of the biggest concerns with Black Box AI is its opacity, we simply don’t know how it reaches its conclusions. Imagine a hospital using an AI system to predict patient mortality rates. If a doctor is told that their patient has “low chances of surviving” and isn’t given any explanation as to why, how can they trust or act on that information?
This lack of interpretability isn’t just frustrating and it can be dangerous. Even leading AI experts recognize this problem. AI researcher Kate Crawford states,
“AI systems are being deployed in high-stakes decisions without clear accountability. We need to understand how they work before we can trust them.”
Transparency matters because it builds trust. Without it, AI feels more like a mystical black box rather than a reliable tool. That’s why researchers are pushing for Explainable AI (XAI), which aims to make AI decisions more interpretable.
2. Ethical and Bias Issues ⚖️
AI is only as fair as the data it learns from and unfortunately, bias is a massive issue. Black Box AI models have been caught reinforcing racial, gender, and socioeconomic biases, often with serious consequences.
Here are some real-world examples:
- Hiring Discrimination – In 2018, an AI-powered hiring tool at Amazon was found to be biased against women, as it had been trained on male-dominated hiring data.
- Racial Bias in Facial Recognition. – Studies by MIT and Georgetown University found that AI-based facial recognition software misidentified Black and Asian individuals at a much higher rate than white individuals
- Unfair Loan Approvals – Some banks’ AI-driven credit scoring systems have been found to discriminate against minority communities, denying them loans despite having similar financial profiles to approved applicants.
Infographic- Statistics on AI Bias Incidents in Different Industries.
Recruitment & HR Amazon’s AI hiring tool favored men over women for tech roles. AI in hiring preferred white candidates over Black candidates 2X more. |
Healthcare A U.S. hospital AI reduced Black patient eligibility by 50% color due to biased training data. AI misdiagnoses skin conditions in people of |
Finance & Lending Black & Hispanic borrowers are 40-80% more likely to be denied loans. AI credit scoring offers lower credit limits to women than men. |
Advertising & Social Media AI showed higher-paying job ads to men over women. Meta’s AI reinforced gender stereotypes in job ads. |
Law Enforcement Facial recognition misidentifies Black & Asian faces 100X more than white faces. AI led to a wrongful arrest in Detroit due to a false match. |
This isn’t just an AI problem, it’s a data problem. If biased historical data is fed into an AI system, the AI learns and amplifies those biases. That’s why organizations must actively audit and refine their AI models to minimize discrimination.
3. Security Risks and Malicious Use
Another rising concern is the potential for Black Box AI to be exploited. Hackers can manipulate AI models through adversarial attacks, where subtle tweaks to inputs cause AI to misclassify data. For instance, a few strategically placed stickers on a stop sign can make an AI-powered self-driving car mistake it for a speed limit sign, a dangerous flaw.
Moreover, bad actors can use Black Box AI for deepfake generation, misinformation campaigns, and automated cyberattacks, making security a growing challenge.
Explainable AI: Shedding Light on the Black Box
Black Box AI may be powerful, but its lack of transparency creates trust issues. This is where Explainable AI (XAI) comes in, it’s an approach designed to make AI decisions more understandable, interpretable, and accountable.
What is Explainable AI (XAI)?
Imagine you're denied a loan by an AI system, but when you ask why, all you get is: "The model decided so." That’s not helpful. XAI aims to fix this by providing clear insights into how AI makes decisions, ensuring that humans can understand, trust, and challenge those decisions when necessary.
According to DARPA (Defense Advanced Research Projects Agency), XAI focuses on creating AI systems that are not only accurate but also interpretable and transparent.
This is especially crucial in high-stakes industries like healthcare, finance, and law, where AI decisions impact real lives. XAI techniques provide insights into how AI models make decisions, enabling users to trust and understand the outcomes.
Why is XAI important?
- Builds trust between humans and AI
- Helps detect and correct biases in AI models
- Ensures compliance with AI regulations (e.g., GDPR’s right to explanation)
- Reduces AI-related risks in sensitive applications like medical diagnoses and legal decisions
Techniques in XAI 🛠️
While traditional AI models operate in a “black box,” XAI introduces various techniques to open the lid and provide explanations. Here are two of the most widely used methods:
1. LIME (Local Interpretable Model-agnostic Explanations)
LIME helps explain individual AI predictions by creating a simpler, interpretable model that mimics the original black-box model for a specific instance.
How LIME Works:
- The AI model makes a prediction (e.g., “This image is a dog”).
- LIME generates a set of similar inputs by slightly modifying the original data.
- It then observes how the model’s prediction changes and identifies the most important features responsible for the decision.
📊 Example: If a medical AI system predicts a high risk of heart disease for a patient, LIME can highlight the key factors influencing the prediction, such as high cholesterol, age, or lifestyle habits.
Flowchart: LIME Interpreting a Black-Box AI Model
Start
↓
Select Instance to Explain
↓
Perturb the Instance
↓
Get Black-Box Predictions
↓
Weight Perturbed Samples
↓
Train Interpretable Model
↓
Extract Feature Importance
↓
Generate Explanation
↓
End
1. Start- Begin the process of interpreting a black-box model.
2. Select Instance to Explain- Choose a specific data instance (e.g., a single row of data) for which you want to generate an explanation.
3. Perturb the Instance- Generate multiple perturbed samples (slightly modified versions) of the selected instance by randomly altering feature values.
4. Get Black-Box Predictions- Use the black-box model to predict the output for each perturbed sample.
5. Weight Perturbed Samples- Assign weights to the perturbed samples based on their proximity to the original instance (e.g., using a distance metric like cosine similarity)
6. Train Interpretable Model- Fit a simple, interpretable model (e.g., linear regression or decision tree) on the perturbed samples and their corresponding black-box predictions. The interpretable model approximates the black-box model locally.
7. Extract Feature Importance- Analyze the coefficients or feature weights of the interpretable model to identify which features contributed most to the black-box model's prediction for the selected instance.
8. Generate Explanation- Present the feature importance scores as an explanation for the black-box model's prediction.
9. End- The process is complete, and the explanation is provided.
2. SHAP (Shapley Additive Explanations)
SHAP, based on game theory, explains how much each feature contributes to an AI model’s output by assigning numerical values (SHAP values) to individual features.
How SHAP Works:
- Each input feature (e.g., income, age, credit history in a loan approval model) is assigned a SHAP value that quantifies its contribution to the final prediction
- These values help visualize which factors had the most influence, making AI decisions more interpretable.
Example: In an AI-driven loan approval system, SHAP can show that:
Feature | SHAP Value (Impact on Approval) |
---|---|
High credit score | +0.35 (positive impact) |
Low income | -0.20 (negative impact) |
Young age | -0.10 (slight negative impact) |
Making AI Decisions More Transparent
By implementing techniques like LIME and SHAP, AI developers and researchers can create models that are both powerful and explainable. The goal of XAI is simple: Make AI decisions as clear and interpretable as human decisions so that users and businesses can trust and rely on AI systems with confidence.
Black Box AI in Coding
AI isn’t just transforming industries like healthcare and finance it’s also revolutionizing the way developers write code. Black Box AI-powered coding tools are making programming faster,
smarter, and more efficient, whether you're a beginner learning Python or an experienced developer working on complex projects.
Tools and Extensions 🛠️
Several AI-powered coding assistants use black box models to provide real-time code suggestions, auto-completions, and even entire code blocks based on simple prompts. One of the most popular tools in this space is Blackbox AI.
What is Blackbox AI Tool?
This tool is a Black Box AI coding assistant that helps developers write, debug, and optimize code with minimal effort. It works similarly to GitHub Copilot and ChatGPT-based coding assistants by analyzing patterns and predicting the next best lines of code.
Key Features of Blackbox AI & Similar Tools:
Feature | Description |
---|---|
Code Suggestions | Predicts and auto-completes code in real time |
Code Chat | Black Box AI chat allows developers to interact with AI for explanations |
Multi-Language Support | Works with Python, JavaScript, C++, and more |
Code Search | Helps find and reuse code from various sources |
Bug Fixing | Bug Fixing Identifies and suggests fixes for errors |
Accessibility: Is Black Box AI Free?
Many AI coding assistants, including Blackbox AI, offer both free and premium plans.
Where Can You Download Blackbox AI?
- Browser Extension: Black Box AI extension is available as a Chrome extension, making it accessible while browsing code repositories.
- VS Code & JetBrains Plugins: Integrated with popular IDEs for a seamless coding experience.
- Standalone Web App: Can be accessed via the Blackbox AI website.
Black Box AI Code Tool: Free vs. Paid Versions
Plan | Features |
---|---|
Free | Basic code suggestions, limited daily queries |
Pro | Advanced code completion, unlimited queries, and debugging assistance |
The Future of AI-Powered Coding
Black Box AI coding assistants aren’t replacing developers they’re enhancing their productivity. These tools help programmers focus on logic rather than syntax, making coding more intuitive and efficient. But with AI-generated code, developers must always verify outputs to ensure accuracy and security.
What’s next?
AI coding assistants will only get smarter, with better natural language understanding, more context awareness, and deeper integration with development workflows. Developers who embrace these tools early will stay ahead of the curve in this rapidly evolving tech landscape. 🚀
Fun Fact-
Some Black Boxes Are Creating Other AI Systems
With neural architecture search (NAS), black box AIs can now design other neural networks better than human engineers.
The Debate: Transparency vs. Performance
As AI becomes more powerful, a critical debate emerges: Should we prioritize transparency or performance in AI models? While explainable AI (XAI) aims to make AI decisions more interpretable, many cutting-edge models especially deep learning systems function like black boxes, delivering high accuracy but minimal transparency.
So, is it possible to have both? Or do we have to sacrifice one for the other? Let’s break it down.
The Balancing Act: Accuracy vs. Interpretability
Factor | Transparent AI (White Box) | Black Box AI |
---|---|---|
Performance | Often lower due to simpler models | High due to complex architectures |
Interpretability | High—easy to understand decisions | Low—decisions are not easily explainable |
Use Cases | Healthcare, finance (where explainability is crucial) | Autonomous systems, large-scale deep learning |
Trust & Ethics | More trusted due to clarity | Less trust due to hidden decision-making |
What Experts Are Saying
AI professionals on LinkedIn, Reddit, and AI research communities are constantly debating this issue. Here’s a look at both sides:
Pro-Transparency Argument
💬 "AI should be explainable, especially in critical sectors like healthcare and criminal justice. If we don’t understand why a model makes a decision, how can we trust it?" – AI Researcher on LinkedIn
Pro-Performance Argument
💬 "The reality is, black box AI models work better. Prioritizing explainability too much could mean losing out on breakthrough innovations." – AI Engineer on Reddit
Can We Have the Best of Both Worlds?
The good news? Researchers are actively working on hybrid models that balance transparency and performance. Explainable AI (XAI) techniques like SHAP and LIME are already helping bridge the gap by offering insights into how models make decisions.
The future of AI may not be about choosing between transparency and performance, but about integrating both into smarter, more responsible AI systems.
Conclusion: Demystifying Black Box AI
AI is advancing at an unprecedented pace, but with it comes the challenge of understanding black box models’ powerful yet opaque systems that shape everything from medical diagnoses to financial decisions.
Throughout this blog, we explored:
✅ What Black Box AI is and how it differs from explainable AI
✅ How it works and where it’s used (healthcare, finance, coding, etc.)
✅ The challenges of transparency, bias, and ethical concerns
✅ Efforts like Explainable AI (XAI) to bridge the gap
✅ The ongoing debate between performance and interpretability
So, where does this leave us? AI is here to stay, but that doesn’t mean we should blindly trust every algorithm. It’s crucial to stay informed, ask questions, and critically assess AI-driven decisions—whether it’s a recommendation system or an autonomous vehicle.
And if you’re serious about understanding and working with AI, now is the time to upskill. Check out this Post Graduate Program in AI to dive deeper into the world of artificial intelligence.
FAQ: Your Questions About Black Box AI
Q 1: Is Black Box AI the same as Machine Learning?
Not exactly! Machine learning is a broad field that includes various AI models, while black box AI refers to models whose decision-making process isn’t easily interpretable. Many deep learning models fall into this category.
Q 2: Can Black Box AI be completely explained?
Not yet. While techniques like LIME and SHAP help interpret AI decisions, fully decoding complex neural networks is still a challenge. That’s why researchers are actively working on Explainable AI (XAI) solutions.
Q 3: Are there real-world risks with Black Box AI?
Absolutely. Imagine an AI-powered loan approval system that denies applications but doesn’t explain why. This lack of transparency can lead to biased decisions, legal issues, and mistrust in AI-driven systems.
Q 4: Is Black Box AI only used for deep learning models?
Mostly, but not exclusively. Deep learning models like neural networks are often black boxes, but even some complex decision trees or ensemble methods can become opaque as they scale.
Q 5: Can I use Black Box AI for coding?
Yes! Tools like Blackbox AI and GitHub Copilot provide AI-powered code suggestions. They enhance productivity but don’t always explain why they suggest certain solutions, so a developer’s critical thinking is still essential.
Q 6: What is Black Box AI Python?
Black Box AI Python refers to the use of Python-based libraries and frameworks (like TensorFlow or Keras) to create deep learning models that are difficult to interpret. These models can make highly accurate predictions but don’t provide clear insights into how they arrive at those decisions.